机器学习 mobi 下载 网盘 caj lrf pdf txt 阿里云
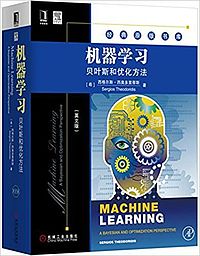
机器学习电子书下载地址
内容简介:
本书对所有主要的机器学习方法和新研究趋势进行了深入探索,涵盖概率和确定性方法以及贝叶斯推断方法。其中,经典方法包括平均/小二乘滤波、卡尔曼滤波、随机逼近和在线学习、贝叶斯分类、决策树、逻辑回归和提升方法等,新趋势包括稀疏、凸分析与优化、在线分布式算法、RKH空间学习、贝叶斯推断、图模型与隐马尔可夫模型、粒子滤波、深度学习、字典学习和潜变量建模等。全书构建了一套明晰的机器学习知识体系,各章内容相对独立,物理推理、数学建模和算法实现精准且细致,并辅以应用实例和习题。本书适合该领域的科研人员和工程师阅读,也适合学习模式识别、统计/自适应信号处理和深度学习等课程的学生参考。
书籍目录:
Contents
Preface.iv
Acknowledgments.vv
Notation.vfivi
CHAPTER 1 Introduction .1
1.1 What Machine Learning is About1
1.1.1 Classification.2
1.1.2 Regression3
1.2 Structure and a Road Map of the Book5
References8
CHAPTER 2 Probability and Stochastic Processes 9
2.1 Introduction.10
2.2 Probability and Random Variables.10
2.2.1Probability11
2.2.2Discrete Random Variables12
2.2.3Continuous Random Variables14
2.2.4Meanand Variance15
2.2.5Transformation of Random Variables.17
2.3 Examples of Distributions18
2.3.1Discrete Variables18
2.3.2Continuous Variables20
2.4 Stochastic Processes29
2.4.1First and Second Order Statistics.30
2.4.2Stationarity and Ergodicity30
2.4.3PowerSpectral Density33
2.4.4Autoregressive Models38
2.5 InformationTheory.41
2.5.1Discrete Random Variables42
2.5.2Continuous Random Variables45
2.6 Stochastic Convergence48
Problems49
References51
CHAPTER 3 Learning in Parametric Modeling: Basic Concepts and Directions 53
3.1 Introduction.53
3.2 Parameter Estimation: The Deterministic Point of View.54
3.3 Linear Regression.57
3.4 Classification60
3.5 Biased Versus Unbiased Estimation.64
3.5.1 Biased or Unbiased Estimation?65
3.6 The Cramér-Rao Lower Bound67
3.7 Suf?cient Statistic.70
3.8 Regularization.72
3.9 The Bias-Variance Dilemma.77
3.9.1 Mean-Square Error Estimation77
3.9.2 Bias-Variance Tradeoff78
3.10 MaximumLikelihoodMethod.82
3.10.1 Linear Regression: The Nonwhite Gaussian Noise Case84
3.11 Bayesian Inference84
3.11.1 The Maximum a Posteriori Probability Estimation Method.88
3.12 Curse of Dimensionality89
3.13 Validation.91
3.14 Expected and Empirical Loss Functions.93
3.15 Nonparametric Modeling and Estimation.95
Problems.97
References102
CHAPTER4 Mean-quare Error Linear Estimation105
4.1Introduction.105
4.2Mean-Square Error Linear Estimation: The Normal Equations106
4.2.1The Cost Function Surface107
4.3A Geometric Viewpoint: Orthogonality Condition109
4.4Extensionto Complex-Valued Variables111
4.4.1Widely Linear Complex-Valued Estimation113
4.4.2Optimizing with Respect to Complex-Valued Variables: Wirtinger Calculus116
4.5Linear Filtering.118
4.6MSE Linear Filtering: A Frequency Domain Point of View120
4.7Some Typical Applications.124
4.7.1Interference Cancellation124
4.7.2System Identification125
4.7.3Deconvolution: Channel Equalization126
4.8Algorithmic Aspects: The Levinson and the Lattice-Ladder Algorithms132
4.8.1The Lattice-Ladder Scheme.137
4.9Mean-Square Error Estimation of Linear Models.140
4.9.1The Gauss-Markov Theorem143
4.9.2Constrained Linear Estimation:The Beamforming Case145
4.10Time-Varying Statistics: Kalman Filtering148
Problems.154
References158
CHAPTER 5 Stochastic Gradient Descent: The LMS Algorithm and its Family .161
5.1 Introduction.162
5.2 The Steepest Descent Method163
5.3 Application to the Mean-Square Error Cost Function167
5.3.1 The Complex-Valued Case175
5.4 Stochastic Approximation177
5.5 The Least-Mean-Squares Adaptive Algorithm179
5.5.1 Convergence and Steady-State Performanceof the LMS in Stationary Environments.181
5.5.2 Cumulative Loss Bounds186
5.6 The Affine Projection Algorithm.188
5.6.1 The Normalized LMS.193
5.7 The Complex-Valued Case.194
5.8 Relatives of the LMS.196
5.9 Simulation Examples.199
5.10 Adaptive Decision Feedback Equalization202
5.11 The Linearly Constrained LMS204
5.12 Tracking Performance of the LMS in Nonstationary Environments.206
5.13 Distributed Learning:The Distributed LMS208
5.13.1Cooperation Strategies.209
5.13.2The Diffusion LMS211
5.13.3 Convergence and Steady-State Performance: Some Highlights218
5.13.4 Consensus-Based Distributed Schemes.220
5.14 A Case Study:Target Localization222
5.15 Some Concluding Remarks: Consensus Matrix.223
Problems.224
References227
CHAPTER 6 The Least-Squares Family 233
6.1 Introduction.234
6.2 Least-Squares Linear Regression: A Geometric Perspective.234
6.3 Statistical Properties of the LS Estimator236
6.4
作者介绍:
作者简介
Sergios Theodoridis 希腊雅典大学信息系教授。主要研究方向是自适应信号处理、通信与模式识别。他是欧洲并行结构及语言协会(PARLE-95)的主席和欧洲信号处理协会(EUSIPCO-98)的常务主席、《信号处理》杂志编委。
Konstantinos Koutroumbas 1995年在希腊雅典大学获得博士学位。自2001年起任职于希腊雅典国家天文台空间应用研究院,是国际知名的专家。
出版社信息:
暂无出版社相关信息,正在全力查找中!
书籍摘录:
暂无相关书籍摘录,正在全力查找中!
原文赏析:
Of course, the author, being human, could not avoid emphasizing the techniques with which he is most familiar. This is healthy, since writing a book is a means of sharing the author's expertise and point of view with readers. This is why I strongly believe that a new book does not come to replace previous ones, but to complement previously published points of view.
For a fixed number of training points, N, in the data sets D, trying to minimize the variance term results in an increase of the bias term and vice versa. This is because, in order to reduce the bias term, one has to increase the complexity (more free parameters) of the adopted estimator f (·; D). This, in turn, results in higher variance as we change the training sets. This is a manifestation of the over fitting issue that we have already discussed. The only way to reduce both terms simultaneously is to increase the number of the training data points, N, and at the same time increase the complexity of the model carefully, so as to achieve the aforementioned goal. If one increases the number of training points and at the same time increases the model complexity excessively, the overall MSE...
其它内容:
书籍介绍
本书对所有主要的机器学习方法和新研究趋势进行了深入探索,涵盖概率和确定性方法以及贝叶斯推断方法。其中,经典方法包括平均/小二乘滤波、卡尔曼滤波、随机逼近和在线学习、贝叶斯分类、决策树、逻辑回归和提升方法等,新趋势包括稀疏、凸分析与优化、在线分布式算法、RKH空间学习、贝叶斯推断、图模型与隐马尔可夫模型、粒子滤波、深度学习、字典学习和潜变量建模等。全书构建了一套明晰的机器学习知识体系,各章内容相对独立,物理推理、数学建模和算法实现精准且细致,并辅以应用实例和习题。本书适合该领域的科研人员和工程师阅读,也适合学习模式识别、统计/自适应信号处理和深度学习等课程的学生参考。
网站评分
书籍多样性:7分
书籍信息完全性:8分
网站更新速度:4分
使用便利性:9分
书籍清晰度:3分
书籍格式兼容性:3分
是否包含广告:9分
加载速度:4分
安全性:4分
稳定性:8分
搜索功能:3分
下载便捷性:5分
下载点评
- 情节曲折(88+)
- 一星好评(441+)
- 三星好评(644+)
- 引人入胜(432+)
- 服务好(278+)
- epub(156+)
- 无水印(162+)
- 值得下载(106+)
- 中评(606+)
下载评价
- 网友 瞿***香:
非常好就是加载有点儿慢。
- 网友 菱***兰:
特好。有好多书
- 网友 冉***兮:
如果满分一百分,我愿意给你99分,剩下一分怕你骄傲
- 网友 郗***兰:
网站体验不错
- 网友 堵***洁:
好用,支持
- 网友 车***波:
很好,下载出来的内容没有乱码。
- 网友 孙***美:
加油!支持一下!不错,好用。大家可以去试一下哦
- 网友 常***翠:
哈哈哈哈哈哈
- 网友 权***颜:
下载地址、格式选择、下载方式都还挺多的
- 网友 蓬***之:
好棒good
- 网友 冯***丽:
卡的不行啊
- 网友 家***丝:
好6666666
- 网友 堵***格:
OK,还可以
喜欢"机器学习"的人也看了
53天天练四年级上册 套装共2册 语文+数学北师大版 2023秋季 mobi 下载 网盘 caj lrf pdf txt 阿里云
变配电设备倒闸操作实例分析 mobi 下载 网盘 caj lrf pdf txt 阿里云
商务英语口语(第二版) mobi 下载 网盘 caj lrf pdf txt 阿里云
2024年广东高职单招普通高中学业水平合格性考试模拟试卷语数英合订本知识点考点专项巩固试卷真题集训练题押题模拟真题配答案解析 mobi 下载 网盘 caj lrf pdf txt 阿里云
电子建设工程预算定额 HYD41-2015 第五册 洁净厂房、数据中心及电子环境工程 mobi 下载 网盘 caj lrf pdf txt 阿里云
全新正版图书 小怪物更爱你的爸爸妈妈 奥德里奇·加西亚图文 浙江摄影出版社 9787551413220 青岛新华书店旗舰店 mobi 下载 网盘 caj lrf pdf txt 阿里云
生命树 mobi 下载 网盘 caj lrf pdf txt 阿里云
高考试题分析(理科综合分册)(2020年版) mobi 下载 网盘 caj lrf pdf txt 阿里云
国际象棋基本技术 其他技术 mobi 下载 网盘 caj lrf pdf txt 阿里云
收录机、AV功放机及影碟机维修入门与提高——电子技术入门与提高丛书 mobi 下载 网盘 caj lrf pdf txt 阿里云
- "十四五"时期商业健康保险发展研究 中国发展出版社 mobi 下载 网盘 caj lrf pdf txt 阿里云
- 专利布局 mobi 下载 网盘 caj lrf pdf txt 阿里云
- 制度才是真正的老板(落地执行版) (3) 金城出版社 mobi 下载 网盘 caj lrf pdf txt 阿里云
- 5G时代的卫星通信 mobi 下载 网盘 caj lrf pdf txt 阿里云
- 小学教材全解三年级下册英语外研版 一年级起点课本适用 mobi 下载 网盘 caj lrf pdf txt 阿里云
- 中级注册安全工程师2019教材+试卷化工专业:安全生产管理+法律法规+技术基础+化工安全技术实务(全8本) mobi 下载 网盘 caj lrf pdf txt 阿里云
- 新版剑桥BEC考试真题详解(4高级剑桥商务英语应试辅导用书) mobi 下载 网盘 caj lrf pdf txt 阿里云
- 独立学派的客体关系理论 mobi 下载 网盘 caj lrf pdf txt 阿里云
- 【九周年店庆回馈】【假一罚万】【当天发】【官方原版全新塑封当天发货】坂茂和他的建筑 纸管木质绿色生态移动集装箱建筑设计 别墅住宅公寓音乐厅展览馆博物馆 mobi 下载 网盘 caj lrf pdf txt 阿里云
- 致艾米丽的秘密手稿 mobi 下载 网盘 caj lrf pdf txt 阿里云
书籍真实打分
故事情节:5分
人物塑造:9分
主题深度:7分
文字风格:7分
语言运用:7分
文笔流畅:6分
思想传递:5分
知识深度:3分
知识广度:9分
实用性:3分
章节划分:3分
结构布局:5分
新颖与独特:4分
情感共鸣:5分
引人入胜:3分
现实相关:6分
沉浸感:5分
事实准确性:5分
文化贡献:5分